Writing views
The fundamentals of a view are straightforward. A view creates a perspective on the data stored in your Couchbase buckets in a format that can be used to represent the data in a specific way, define and filter the information, and provide a basis for searching or querying the data in the database based on the content. During the view creation process, you define the output structure, field order, content and any summary or grouping information desired in the view.
Views achieve this by defining an output structure that translates the stored JSON object data into a JSON array or object across two components, the key and the value. This definition is performed through the specification of two separate functions written in JavaScript. The view definition is divided into two parts, a map function and a reduce function:
- Map function
As the name suggests, the map function creates a mapping between the input data (the JSON objects stored in your database) and the data as you want it displayed in the results (output) of the view. Every document in the Couchbase bucket for the view is submitted to the map() function in each view once, and it is the output from the map() function that is used as the result of the view.
The map() function is supplied two arguments by the views processor. The first argument is the JSON document data. The optional second argument is the associated metadata for the document, such as the expiration, flags, and revision information.
The map function outputs zero or more ‘rows’ of information using an emit() function. Each call to the emit() function is equivalent to a row of data in the view result. The emit() function can be called multiple times within the single pass of the map() function. This functionality enables you to create views that may expose information stored in a compound format within a single stored JSON record, for example generating a row for each item in an array.
You can see this in the figure below, where the name, salary and city fields of the stored JSON documents are translated into a table (an array of fields) in the generated view content.
- Reduce function
The reduce function is used to summarize the content generated during the map phase. Reduce functions are optional in a view and do not have to be defined. When they exist, each row of output (from each emit() call in the corresponding map() function) is processed by the corresponding reduce() function.
If a reduce function is specified in the view definition it is automatically used. You can access a view without enabling the reduce function by disabling reduction ( reduce=false ) when the view is accessed.
Typical uses for a reduce function are to produce a summarized count of the input data, or to provide sum or other calculations on the input data. For example, if the input data included employee and salary data, the reduce function could be used to produce a count of the people in a specific location, or the total of all the salaries for people in those locations.
The combination of the map and the reduce function produce the corresponding view. The two functions work together, with the map producing the initial material based on the content of each JSON document, and the reduce function summarizing the information generated during the map phase. The reduction process is selectable at the point of accessing the view, you can choose whether to the reduce the content or not, and, by using an array as the key, you can specifying the grouping of the reduce information.
Each row in the output of a view consists of the view key and the view value. When accessing a view using only the map function, the contents of the view key and value are those explicitly stated in the definition. In this mode the view will also always contain an id field which contains the document ID of the source record (i.e. the string used as the ID when storing the original data record).
When accessing a view employing both the map and reduce functions the key and value are derived from the output of the reduce function based on the input key and group level specified. A document ID is not automatically included because the document ID cannot be determined from reduced data where multiple records may have been merged into one. Examples of the different explicit and implicit values in views will be shown as the details of the two functions are discussed.
You can see an example of the view creation process in the figure below.
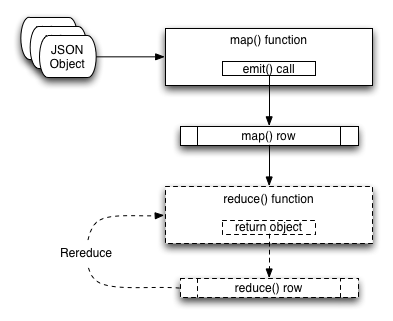
Because of the separation of the two elements, you can consider the two functions individually.
For information on how to write map functions, and how the output of the map function affects and supports searching.
View names must be specified using one or more UTF–8 characters. You cannot have a blank view name. View names cannot have leading or trailing whitespace characters (space, tab, newline, or carriage-return).
To create views, you can use either the Admin Console View editor, use the REST API for design documents, or use one of the client libraries that support view management.
Map functions
The map function is the most critical part of any view as it provides the logical mapping between the input fields of the individual objects stored within Couchbase to the information output when the view is accessed.
Through this mapping process, the map function and the view provide:
The output format and structure of the view on the bucket.
Structure and information used to query and select individual documents using the view information.
Sorting of the view results.
Input information for summarizing and reducing the view content.
Applications access views through the REST API, or through a Couchbase client library. All client libraries provide a method for submitting a query into the view system and obtaining and processing the results.
The basic operation of the map function can be seen in the figure below.
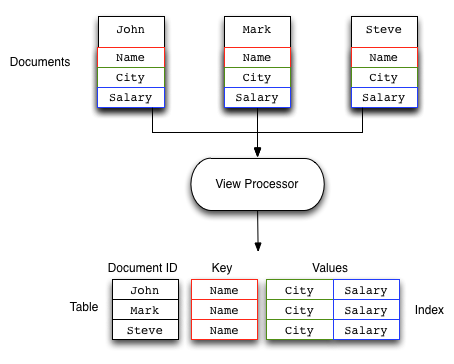
In this example, a map function is taking the Name, City, and Salary fields from the JSON documents stored in the Couchbase bucket and mapping them to a table of these fields. The map function which produces this output might look like this:
function(doc, meta)
{
emit(doc.name, [doc.city, doc.salary]);
}
When the view is generated the map() function is supplied two arguments for each stored document, doc and meta :
- doc
The stored document from the Couchbase bucket, either the JSON or binary content. Content type can be identified by accessing the type field of the meta argument object.
- meta
The metadata for the stored document, containing expiry time, document ID, revision and other information.
Every document in the Couchbase bucket is submitted to the map() function in turn. After the view is created, only the documents created or changed since the last update need to be processed by the view. View indexes and updates are materialized when the view is accessed. Any documents added or changed since the last access of the view will be submitted to the map() function again so that the view is updated to reflect the current state of the data bucket.
Within the map() function itself you can perform any formatting, calculation or other detail. To generate the view information, you use calls to the emit() function. Each call to the emit() function outputs a single row or record in the generated view content.
The emit() function accepts two arguments, the key and the value for each record in the generated view:
- key
The emitted key is used by Couchbase Server both for sorting and querying the content in the database.
The key can be formatted in a variety of ways, including as a string or compound value (such as an array or JSON object). The content and structure of the key is important, because it is through the emitted key structure that information is selected within the view.
All views are output in a sorted order according to the content and structure of the key. Keys using a numeric value are sorted numerically, for strings, UTF–8 is used. Keys can also support compound values such as arrays and hashes.
The key content is used for querying by using a combination of this sorting process and the specification of either an explicit key or key range within the query specification. For example, if a view outputs the RECIPE TITLE field as a key, you could obtain all the records matching ‘Lasagne’ by specifying that only the keys matching ‘Lasagne’ are returned.
- value
The value is the information that you want to output in each view row. The value can be anything, including both static data, fields from your JSON objects, and calculated values or strings based on the content of your JSON objects.
The content of the value is important when performing a reduction, since it is the value that is used during reduction, particularly with the built-in reduction functions. For example, when outputting sales data, you might put the SALESMAN into the emitted key, and put the sales amounts into the value. The built-in _sum function will then total up the content of the corresponding value for each unique key.
The format of both key and value is up to you. You can format these as single values, strings, or compound values such as arrays or JSON. The structure of the key is important because you must specify keys in the same format as they were generated in the view specification.
The emit() function can be called multiple times in a single map function, with each call outputting a single row in the generated view. This can be useful when you want to supporting querying information in the database based on a compound field. For a sample view definition and selection criteria.
Views and map generation are also very forgiving. If you elect to output fields in from the source JSON objects that do not exist, they will simply be replaced with a null value, rather than generating an error.
For example, in the view below, some of the source records do contain all of the fields in the specified view. The result in the view result is just the null entry for that field in the value output.
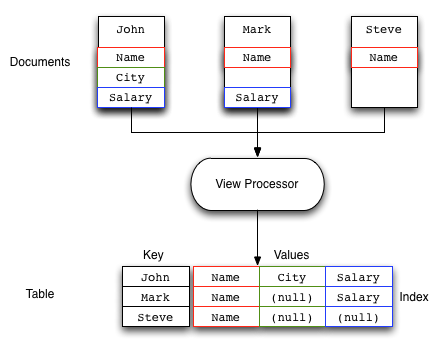
You should check that the field or data source exists during the map processing before emitting the data.
To better understand how the map function works to output different types of information and retrieve it, see View and Query Pattern Samples.
Reduce functions
Often the information that you are searching or reporting on needs to be summarized or reduced. There are a number of different occasions when this can be useful. For example, if you want to obtain a count of all the items of a particular type, such as comments, recipes matching an ingredient, or blog entries against a keyword.
When using a reduce function in your view, the value that you specify in the call to emit() is replaced with the value generated by the reduce function. This is because the value specified by emit() is used as one of the input parameters to the reduce function. The reduce function is designed to reduce a group of values emitted by the corresponding map() function.
Alternatively, reduce can be used for performing sums, for example totalling all the invoice values for a single client, or totalling up the preparation and cooking times in a recipe. Any calculation that can be performed on a group of the emitted data.
In each of the above cases, the raw data is the information from one or more rows of information produced by a call to emit(). The input data, each record generated by the emit() call, is reduced and grouped together to produce a new record in the output.
The grouping is performed based on the value of the emitted key, with the rows of information generated during the map phase being reduced and collated according to the uniqueness of the emitted key.
When using a reduce function the reduction is applied as follows:
- For each record of input, the corresponding reduce function is applied on the row, and the return value from the reduce function is the resulting row.
For example, using the built-in _sum reduce function, the value in each case would be totaled based on the emitted key:
```
{
"rows" : [
{"value" : 13000, "id" : "James", "key" : "James" },
{"value" : 20000, "id" : "James", "key" : "James" },
{"value" : 5000, "id" : "Adam", "key" : "Adam" },
{"value" : 8000, "id" : "Adam", "key" : "Adam" },
{"value" : 10000, "id" : "John", "key" : "John" },
{"value" : 34000, "id" : "John", "key" : "John" }
]
}
```
Using the unique key of the name, the data generated by the map above would be reduced, using the key as the collator, to the produce the following output:
{
"rows" : [
{"value" : 33000, "key" : "James" },
{"value" : 13000, "key" : "Adam" },
{"value" : 44000, "key" : "John" },
]
}
In each case the values for the common keys (John, Adam, James), have been totalled, and the six input rows reduced to the 3 rows shown here.
Results are grouped on the key from the call to emit() if grouping is selected during query time. As shown in the previous example, the reduction operates by the taking the key as the group value as using this as the basis of the reduction.
If you use an array as the key, and have selected the output to be grouped during querying you can specify the level of the reduction function, which is analogous to the element of the array on which the data should be grouped.
The view definition is flexible. You can select whether the reduce function is applied when the view is accessed. This means that you can access both the reduced and unreduced (map-only) content of the same view. You do not need to create different views to access the two different types of data.
Whenever the reduce function is called, the generated view content contains the same key and value fields for each row, but the key is the selected group (or an array of the group elements according to the group level), and the value is the computed reduction value.
Couchbase includes the following built-in reduce functions:
- _count
- _sum
- _stats.
The reduce function also has a final additional benefit. The results of the computed reduction are stored in the index along with the rest of the view information. This means that when accessing a view with the reduce function enabled, the information comes directly from the index content. This results in a very low impact on the Couchbase Server to the query (the value is not computed at runtime), and results in very fast query times, even when accessing information based on a range-based query.
The reduce() function is designed to reduce and summarize the data emitted during the map() phase of the process. It should only be used to summarize the data, and not to transform the output information or concatenate the information into a single structure.
When using a composite structure, the size limit on the composite structure within the reduce() function is 64KB.
Built-in _count
The _count function provides a simple count of the input rows from the map() function, using the keys and group level to provide a count of the correlated items. The values generated during the map() stage are ignored.
For example, using the input:
{
"rows" : [
{"value" : 13000, "id" : "James", "key" : ["James", "Paris"] },
{"value" : 20000, "id" : "James", "key" : ["James", "Tokyo"] },
{"value" : 5000, "id" : "James", "key" : ["James", "Paris"] },
{"value" : 7000, "id" : "Adam", "key" : ["Adam", "London"] },
{"value" : 19000, "id" : "Adam", "key" : ["Adam", "Paris"] },
{"value" : 17000, "id" : "Adam", "key" : ["Adam", "Tokyo"] },
{"value" : 22000, "id" : "John", "key" : ["John", "Paris"] },
{"value" : 3000, "id" : "John", "key" : ["John", "London"] },
{"value" : 7000, "id" : "John", "key" : ["John", "London"] },
]
}
Enabling the reduce() function and using a group level of 1 would produce:
{
"rows" : [
{"value" : 3, "key" : ["Adam" ] },
{"value" : 3, "key" : ["James"] },
{"value" : 3, "key" : ["John" ] }
]
}
The reduction has produce a new result set with the key as an array based on the first element of the array from the map output. The value is the count of the number of records collated by the first element.
Using a group level of 2 would generate the following:
{
"rows" : [
{"value" : 1, "key" : ["Adam", "London"] },
{"value" : 1, "key" : ["Adam", "Paris" ] },
{"value" : 1, "key" : ["Adam", "Tokyo" ] },
{"value" : 2, "key" : ["James","Paris" ] },
{"value" : 1, "key" : ["James","Tokyo" ] },
{"value" : 2, "key" : ["John", "London"] },
{"value" : 1, "key" : ["John", "Paris" ] }
]
}
Now the counts are for the keys matching both the first two elements of the map output.
Built-in _sum
The built-in _sum function sums the values from the map() function call, this time summing up the information in the value for each row. The information can either be a single number or during a rereduce an array of numbers.
The input values must be a number, not a string-representation of a number. The entire map/reduce will fail if the reduce input is not in the correct format. You should use the parseInt() or parseFloat() function calls within your map() function stage to ensure that the input data is a number.
For example, using the same sales source data, accessing the group level 1 view would produce the total sales for each salesman:
{
"rows" : [
{"value" : 43000, "key" : [ "Adam" ] },
{"value" : 38000, "key" : [ "James" ] },
{"value" : 32000, "key" : [ "John" ] }
]
}
Using a group level of 2 you get the information summarized by salesman and city:
{
"rows" : [
{"value" : 7000, "key" : [ "Adam", "London" ] },
{"value" : 19000, "key" : [ "Adam", "Paris" ] },
{"value" : 17000, "key" : [ "Adam", "Tokyo" ] },
{"value" : 18000, "key" : [ "James", "Paris" ] },
{"value" : 20000, "key" : [ "James", "Tokyo" ] },
{"value" : 10000, "key" : [ "John", "London" ] },
{"value" : 22000, "key" : [ "John", "Paris" ] }
]
}
Built-in _stats
The built-in _stats reduce function produces statistical calculations for the input data. As with the _sum function, the corresponding value in the emit call should be a number. The generated statistics include the sum, count, minimum ( min ), maximum ( max ) and sum squared ( sumsqr ) of the input rows.
Using the sales data, a slightly truncated output at group level one would be:
{
"rows" : [
{
"value" : {
"count" : 3,
"min" : 7000,
"sumsqr" : 699000000,
"max" : 19000,
"sum" : 43000
},
"key" : [
"Adam"
]
},
{
"value" : {
"count" : 3,
"min" : 5000,
"sumsqr" : 594000000,
"max" : 20000,
"sum" : 38000
},
"key" : [
"James"
]
},
{
"value" : {
"count" : 3,
"min" : 3000,
"sumsqr" : 542000000,
"max" : 22000,
"sum" : 32000
},
"key" : [
"John"
]
}
]
}
The same fields in the output value are provided for each of the reduced output rows.
Writing custom reduce functions
The reduce() function has to work slightly differently to the map() function. In the primary form, a reduce() function must convert the data supplied to it from the corresponding map() function.
The core structure of the reduce function execution is shown the figure below.
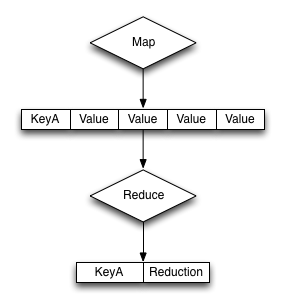
The base format of the reduce() function is as follows:
function(key, values, rereduce) {
…
return retval;
}
The reduce function is supplied three arguments:
- key
The key is the unique key derived from the map() function and the group_level parameter.
- values
The values argument is an array of all of the values that match a particular key. For example, if the same key is output three times, data will be an array of three items containing, with each item containing the value output by the emit() function.
- rereduce
The rereduce indicates whether the function is being called as part of a re-reduce, that is, the reduce function being called again to further reduce the input data.
When rereduce is false:
* The supplied `key` argument will be an array where the first argument is the
`key` as emitted by the map function, and the `id` is the document ID that
generated the key.
* The values is an array of values where each element of the array matches the
corresponding element within the array of `keys`.
When rereduce is true:
* `key` will be null.
* `values` will be an array of values as returned by a previous `reduce()`
function.
The function should return the reduced version of the information by calling the return() function. The format of the return value should match the format required for the specified key.
Re-writing the built-in reduce functions
Using this model as a template, it is possible to write the full implementation of the built-in functions _sum and _count when working with the sales data and the standard map() function below:
function(doc, meta)
{
emit(meta.id, null);
}
The _count function returns a count of all the records for a given key. Since argument for the reduce function contains an array of all the values for a given key, the length of the array needs to be returned in the reduce() function:
function(key, values, rereduce) {
if (rereduce) {
var result = 0;
for (var i = 0; i < values.length; i++) {
result += values[i];
}
return result;
} else {
return values.length;
}
}
To explicitly write the equivalent of the built-in _sum reduce function, the sum of supplied array of values needs to be returned:
function(key, values, rereduce) {
var sum = 0;
for(i=0; i < values.length; i++) {
sum = sum + values[i];
}
return(sum);
}
In the above function, the array of data values is iterated over and added up, with the final value being returned.
Handling re-reduce
For reduce() functions, they should be both transparent and standalone. For example, the _sum function did not rely on global variables or parsing of existing data, and didn’t need to call itself, hence it is also transparent.
In order to handle incremental map/reduce functionality (i.e. updating an existing view), each function must also be able to handle and consume the functions own output. This is because in an incremental situation, the function must be handle both the new records, and previously computed reductions.
This can be explicitly written as follows:
f(keys, values) = f(keys, [ f(keys, values) ])
This can been seen graphically in the illustration below, where previous reductions are included within the array of information are re-supplied to the reduce function as an element of the array of values supplied to the reduce function.
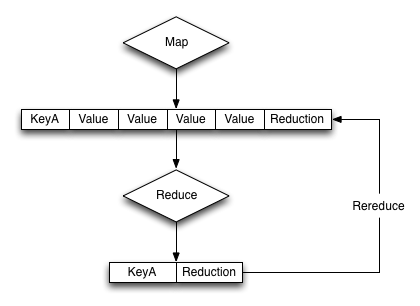
That is, the input of a reduce function can be not only the raw data from the map phase, but also the output of a previous reduce phase. This is called rereduce, and can be identified by the third argument to the reduce(). When the rereduce argument is true, both the key and values arguments are arrays, with the corresponding element in each containing the relevant key and value. I.e., key[1] is the key related to the value of value[1].
An example of this can be seen by considering an expanded version of the sum function showing the supplied values for the first iteration of the view index building:
function('James', [ 13000,20000,5000 ]) {...}
When a document with the ‘James’ key is added to the database, and the view operation is called again to perform an incremental update, the equivalent call is:
function('James', [ 19000, function('James', [ 13000,20000,5000 ]) ]) { ... }
In reality, the incremental call is supplied the previously computed value, and the newly emitted value from the new document:
function('James', [ 19000, 38000 ]) { ... }
Fortunately, the simplicity of the structure for sum means that the function both expects an array of numbers, and returns a number, so these can easily be recombined.
If writing more complex reductions, where a compound key is output, the reduce() function must be able to handle processing an argument of the previous reduction as the compound value in addition to the data generated by the map() phase. For example, to generate a compound output showing both the total and count of values, a suitable reduce() function could be written like this:
function(key, values, rereduce) {
var result = {total: 0, count: 0};
for(i=0; i < values.length; i++) {
if(rereduce) {
result.total = result.total + values[i].total;
result.count = result.count + values[i].count;
} else {
result.total = sum(values);
result.count = values.length;
}
}
return(result);
}
Each element of the array supplied to the function is checked using the built-in typeof function to identify whether the element was an object (as output by a previous reduce), or a number (from the map phase), and then updates the return value accordingly.
Using the sample sales data, and group level of two, the output from a reduced view may look like this:
{"rows":[
{"key":["Adam", "London"],"value":{"total":7000, "count":1}},
{"key":["Adam", "Paris"], "value":{"total":19000, "count":1}},
{"key":["Adam", "Tokyo"], "value":{"total":17000, "count":1}},
{"key":["James","Paris"], "value":{"total":118000,"count":3}},
{"key":["James","Tokyo"], "value":{"total":20000, "count":1}},
{"key":["John", "London"],"value":{"total":10000, "count":2}},
{"key":["John", "Paris"], "value":{"total":22000, "count":1}}
]
}
Reduce functions must be written to cope with this scenario in order to cope with the incremental nature of the view and index building. If this is not handled correctly, the index will fail to be built correctly.
The reduce() function is designed to reduce and summarize the data emitted during the map() phase of the process. It should only be used to summarize the data, and not to transform the output information or concatenate the information into a single structure.
When using a composite structure, the size limit on the composite structure within the reduce() function is 64KB.
Views on non-JSON data
If the data stored within your buckets is not JSON formatted or JSON in nature, then the information is stored in the database as an attachment to a JSON document returned by the core database layer.
This does not mean that you cannot create views on the information, but it does limit the information that you can output with your view to the information exposed by the document key used to store the information.
At the most basic level, this means that you can still do range queries on the key information. For example:
function(doc, meta)
{
emit(meta.id, null);
}
You can now perform range queries by using the emitted key data and an appropriate startkey and endkey value.
If you use a structured format for your keys, for example using a prefix for the data type, or separators used to identify different elements, then your view function can output this information explicitly in the view. For example, if you use a key structure where the document ID is defined as a series of values that are colon separated:
OBJECTYPE:APPNAME:OBJECTID
You can parse this information within the JavaScript map/reduce query to output each item individually. For example:
function(doc, meta)
{
values = meta.id.split(':',3);
emit([values[0], values[1], values[2]], null);
}
The above function will output a view that consists of a key containing the object type, application name, and unique object ID. You can query the view to obtain all entries of a specific object type using:
startkey=['monster', null, null]&endkey=['monster','\u0000' ,'\u0000']
Built-in utility functions
Couchbase Server incorporates different utility function beyond the core JavaScript functionality that can be used within map() and reduce() functions where relevant.
- dateToArray(date)
Converts a JavaScript Date object or a valid date string such as “2012–07–30T23:58:22.193Z” into an array of individual date components. For example, the previous string would be converted into a JavaScript array:
```
[2012, 7, 30, 23, 58, 22]
```
The function can be particularly useful when building views using dates as the key where the use of a reduce function is being used for counting or rollup.
Currently, the function works only on UTC values. Timezones are not supported.
- decodeBase64(doc)
Converts a binary (base64) encoded value stored in the database into a string. This can be useful if you want to output or parse the contents of a document that has not been identified as a valid JSON value.
- sum(array)
When supplied with an array containing numerical values, each value is summed and the resulting total is returned.
For example:
sum([12,34,56,78])
View writing best practice
Although you are free to write views matching your data, you should keep in mind the performance and storage implications of creating and organizing the different design document and view definitions.
You should keep the following in mind while developing and deploying your views:
- Quantity of Views per Design Document
Because the index for each map/reduce combination within each view within a given design document is updated at the same time, avoid declaring too many views within the same design document. For example, if you have a design document with five different views, all five views will be updated simultaneously, even if only one of the views is accessed.
This can result in increase view index generation times, especially for frequently accessed views. Instead, move frequently used views out to a separate design document.
The exact number of views per design document should be determined from a combination of the update frequency requirements on the included views and grouping of the view definitions. For example, if you have a view that needs to be updated with a high frequency (for example, comments on a blog post), and another view that needs to be updated less frequently (e.g. top blogposts), separate the views into two design documents so that the comments view can be updated frequently, and independently, of the other view.
You can always configure the updating of the view through the use of the stale parameter. You can also configure different automated view update times for individual design documents
- Modifying Existing Views
If you modify an existing view definition, or are executing a full build on a development view, the entire view will need to be recreated. In addition, all the views defined within the same design document will also be recreated.
Rebuilding all the views within a single design document is an expensive operation in terms of I/O and CPU requirements, as each document will need to be parsed by each views map() and reduce() functions, with the resulting index stored on disk.
This process of rebuilding will occur across all the nodes within the cluster and increases the overall disk I/O and CPU requirements until the view has been recreated. This process will take place in addition to any production design documents and views that also need to be kept up to date.
- Don’t Include Document ID
The document ID is automatically output by the view system when the view is accessed. When accessing a view without reduce enabled you can always determine the document ID of the document that generated the row. You should not include the document ID (from meta.id ) in your key or value data.
- Check Document Fields
Fields and attributes from source documentation in map() or reduce() functions should be checked before their value is checked or compared. This can cause issues because the view definitions in a design document are processed at the same time. A common cause of runtime errors in views is missing or invalid field and attribute checking.
The most common issue is a field within a null object being accessed. This generates a runtime error that will cause execution of all views within the design document to fail. To address this problem, you should check for the existence of a given object before it is used, or the content value is checked. For example, the following view will fail if the doc.ingredient object does not exist, because accessing the length attribute on a null object will fail:
```
function(doc, meta)
{
emit(doc.ingredient.ingredtext, null);
}
```
Adding a check for the parent object before calling emit() ensures that the function is not called unless the field in the source document exists:
```
function(doc, meta)
{
if (doc.ingredient)
{
emit(doc.ingredient.ingredtext, null);
}
}
```
The same check should be performed when comparing values within the if statement.
This test should be performed on all objects where you are checking the attributes or child values (for example, indices of an array).
- View Size, Disk Storage and I/O
Within the map function, the information declared within your emit() statement is included in the view index data and stored on disk. Outputting this information will have the following effects on your indexes:
* *Increased index size on disk* — More detailed or complex key/value combinations
in generated views will result in more information being stored on disk.
* *Increased disk I/O* — in order to process and store the information on disk,
and retrieve the data when the view is queried. A larger more complex key/value
definition in your view will increase the overall disk I/O required both to
update and read the data back.
The result is that the index can be quite large, and in some cases, the size of the index can exceed the size of the original source data by a significant factor if multiple views are created, or you include large portions or the entire document data in the view output.
For example, if each view contains the entire document as part of the value, and you define ten views, the size of your index files will be more than 10 times the size of the original data on which the view was created. With a 500-byte document and 1 million documents, the view index would be approximately 5GB with only 500MB of source data.
- Including Value Data in Views
Views store both the key and value emitted by the emit(). To ensure the highest performance, views should only emit the minimum key data required to search and select information. The value output by emit() should only be used when you need the data to be used within a reduce().
You can obtain the document value by using the core Couchbase API to get individual documents or documents in bulk. Some SDKs can perform this operation for you automatically.
Using this model will also prevent issues where the emitted view data may be inconsistent with the document state and your view is emitting value data from the document which is no longer stored in the document itself.
For views that are not going to be used with reduce, you should output a null value:
```
function(doc, meta)
{
if(doc.type == 'object')
emit(doc.experience, null);
}
```
This will create an optimized view containing only the information required, ensuring the highest performance when updating the view, and smaller disk usage.
- Don’t Include Entire Documents in View output
A view index should be designed to provide base information and through the implicitly returned document ID point to the source document. It is bad practice to include the entire document within your view output.
You can always access the full document data through the client libraries by later requesting the individual document data. This is typically much faster than including the full document data in the view index, and enables you to optimize the index performance without sacrificing the ability to load the full document data.
For example, the following is an example of a bad view:
```
function(doc, meta)
{
if(doc.type == 'object')
emit(doc.experience, doc);
}
```
The above view may have significant performance and index size effects.
This will include the full document content in the index.
Instead, the view should be defined as:
```
function(doc, meta)
{
if(doc.type == 'object')
emit(doc.experience, null);
}
```
You can then either access the document data individually through the client libraries, or by using the built-in client library option to separately obtain the document data.
- Using Document Types
If you are using a document type (by using a field in the stored JSON to indicate the document structure), be aware that on a large database this can mean that the view function is called to update the index for document types that are not being updated or added to the index.
For example, within a database storing game objects with a standard list of objects, and the users that interact with them, you might use a field in the JSON to indicate ‘object’ or ‘player’. With a view that outputs information when the document is an object:
```
function(doc, meta)
{
emit(doc.experience, null);
}
```
If only players are added to the bucket, the map/reduce functions to update this view will be executed when the view is updated, even though no new objects are being added to the database. Over time, this can add a significant overhead to the view building process.
In a database organization like this, it can be easier from an application perspective to use separate buckets for the objects and players, and therefore completely separate view index update and structure without requiring to check the document type during progressing.
- Use Built-in Reduce Functions
Where possible, use one of the supplied built-in reduce functions, _sum, _count](#couchbase-views-writing-reduce-count), _stats](#couchbase-views-writing-reduce-stats).
These functions are highly optimized. Using a custom reduce function requires additional processing and may impose additional build time on the production of the index.